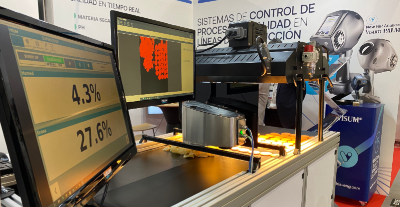
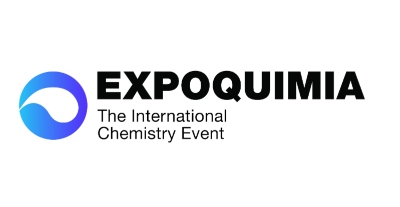
New Visum Palm™ AI-assisted handheld NIR analyser
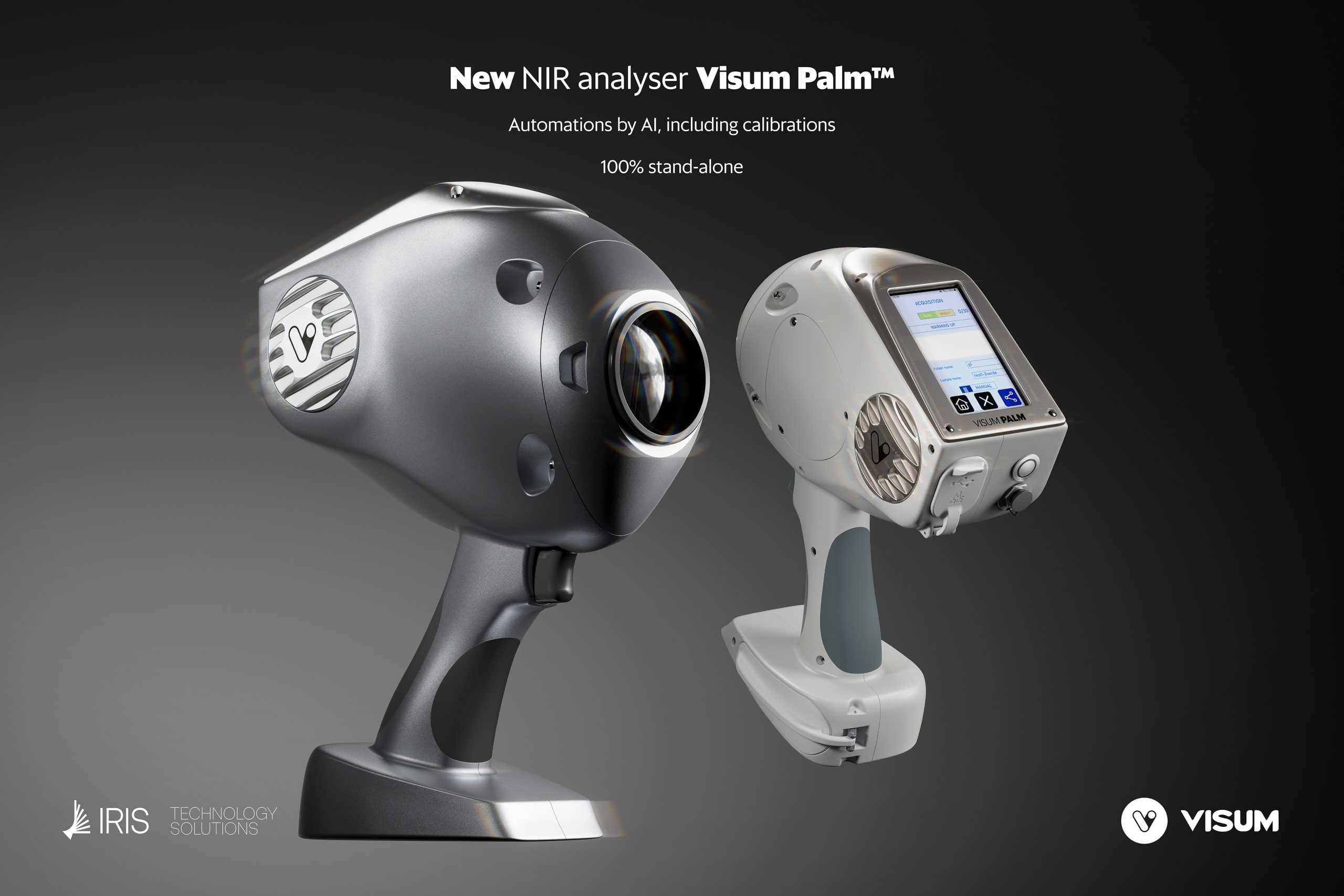
New Visum Palm™ AI-assisted handheld NIR analyser
IRIS Technology Solutions introduces the latest version of its Visum Palm™ portable NIR analyser to complement its Visum® range of real-time process analysers for industry.
The new Visum Palm™ is a fully portable NIR spectrophotometer that allows real-time analysis of different substances, products or mixtures, without the need for traditional laboratory and sampling techniques, allowing industry to obtain results on the spot to make decisions or correct production process parameters.
The new generation Visum Palm™ brings with it an innovative design and a radical change in the way users experience NIR technology, now assisted by AI with the Visum Master™ software, so that each manufacturer can automatically create their own predictive models or calibrations according to their control and analysis needs.
Design, autonomy and robustness
The Visum Palm™ handheld nir analyser offers an innovative and ergonomic design, as well as the possibility to perform analysis at any time and place without having to connect it to any external electronic device. This is possible because it incorporates an embedded touch screen and computer, which enable all the routine functionalities of the device.
The Visum Palm™ operates in the 900 to 1700 nm range, as this is the band that best combines availability of chemical information with price and technological maturity. It operates mainly in diffuse reflectance mode, for which it has specially designed and patented optics to extract as much information as possible from the sample. Specifically, it has a large illumination area (50 mm diameter) and a collection area of 10 mm. These features differentiate it from similar analysers in terms of its suitability for analysing heterogeneous samples, which is most often the case in real working conditions. In cases where heterogeneity is more evident, the device is configurable to calculate and report the average of a given number of repetitions.
The Visum Palm™ handheld NIR analyser is IP65 compliant, making it resistant to dust, moisture and water. It is also rugged enough to be carried and tested almost anywhere indoors or outdoors and even comes with a stand for desktop or tabletop use.
A new AI-assisted user experience
Unlike most common modelling and calibration software on the market, which requires the user to have some technical knowledge of chemometrics or entrust the task to a third party, Visum Master™ PC-based software makes NIR technology even more accessible by automating pre-processing, multivariate analysis algorithm selection and validation. This allows any user to generate models by simply inputting spectra and references (quantitative or qualitative) for routine real-time analysis to replace traditional analysis.
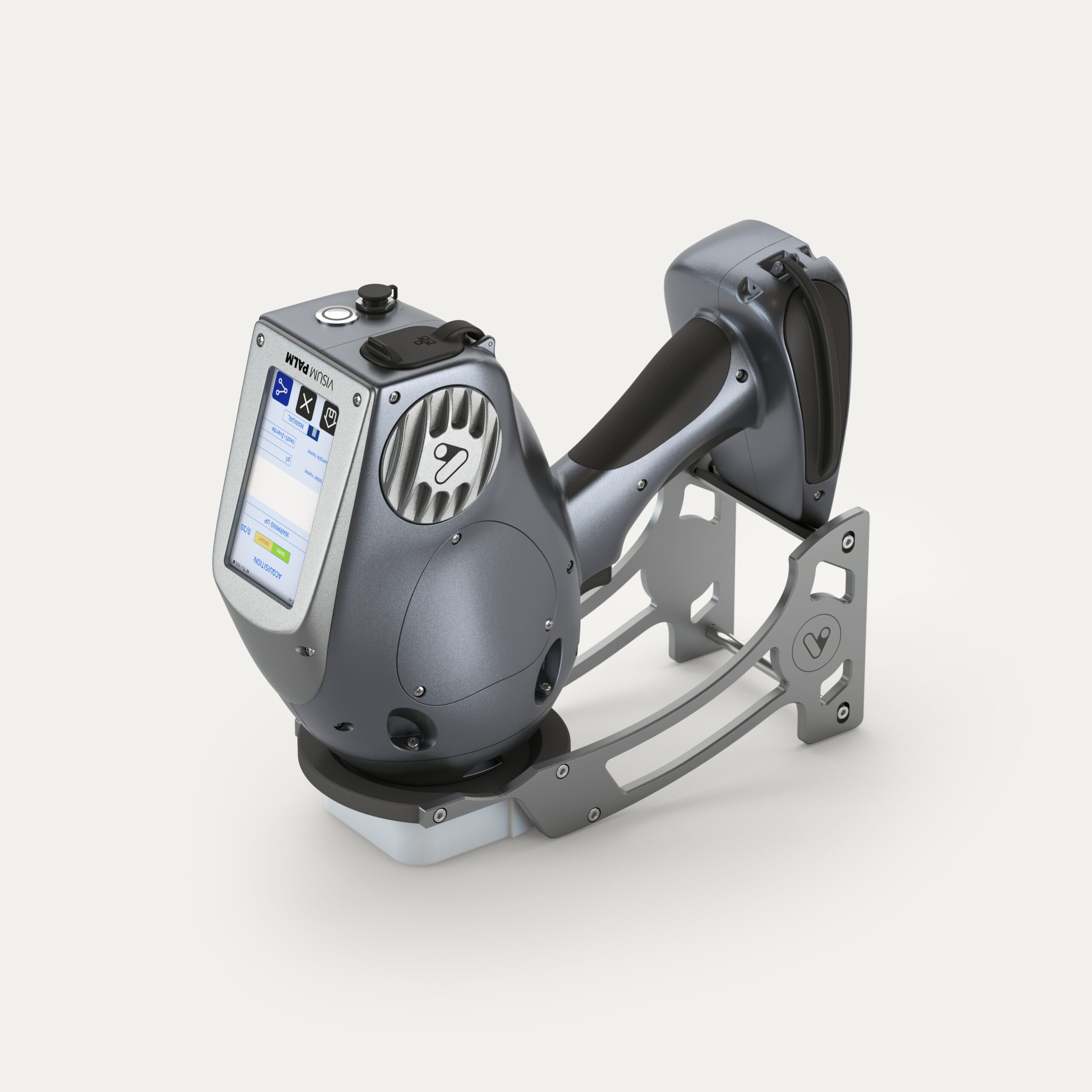
The new software also allows to extend and edit pre-existing models, synchronise with the portable analyser to import spectra, export models, download measurement results, automatically generate analytical method validation reports and audit reports for GMP environments, and to check the metrological performance of the device in a guided manner when needed.
For industry and GMP environments
While NIR technology has a myriad of applications in numerous industries such as plastics, food, chemical, agribusiness, wood, biofuels, to mention the most relevant but not the only ones; it is for the pharmaceutical industry and GMP environments where the new Visum Palm™ device introduces significant novelties at the level of usability and functionality. It is 21 CFR Part 11 compliant, allowing the generation and display of an automatic Audit Trail report, the record of all device activity, where comments and observations can be incorporated. It also allows the user to automatically generate the analytical method validations developed and perform metrological checks of the device when required and download the results at a later date.
“NIR technology today must be easy to use and understand, and at the same time it must give the user the freedom and autonomy to exploit it to the full and facilitate their day-to-day work. Technology must be an enabler. We will continue to take further steps in terms of automation and new functionalities because we are convinced that this is the right way forward and what the industry and the people in it need”, says Oonagh Mc Nerney, Director of IRIS Technology Solutions, S.L.
The new Visum Palm™ handheld NIR analyser is now available here, where you can also find technical information about the device, videos and contact IRIS Technology Solutions, S.L. for a demonstration or specific enquiry.